Machine Learning as a Source of Good: Empowering Nonprofits Through Data-Driven Innovation
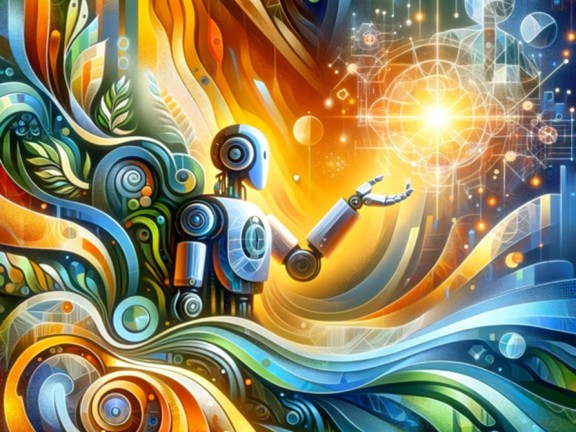
Author: Kamran Makarian
In today's data-centric world, nonprofits stand at a crossroads of unprecedented opportunity. Machine learning (ML), a subset of artificial intelligence (AI), presents a transformative toolkit to harness data for social good. For decision-makers in the nonprofit sector, understanding and implementing ML is not just innovative – it's imperative for increasing impact. This guide aims to explain ML, explore its applications, and offer insights into its ethical development.
Nonprofits often have unique data environments and operational constraints, requiring a customized approach to ML implementation. For instance, a nonprofit focused on homelessness might leverage predictive models to identify at-risk populations by integrating data from various social services. By contrast, an environmental organization could use image recognition models to track deforestation trends from satellite imagery. However, when integrating ML, it is crucial to evaluate existing digital infrastructure.
Many nonprofits might already use a customer relationship management (CRM) system, which can be a data source for ML models. For example, an ML model could analyze donation patterns within the CRM to forecast fundraising trends and segment donors, tailoring communication strategies accordingly. The technical process involves data extraction, cleaning, and preprocessing to ensure the ML model receives high-quality input. Then, model selection is performed based on the nonprofit's specific goals, followed by training the model with relevant data. This integration demands not only technical expertise but also strategic planning to align the ML capabilities with the mission-driven objectives of the nonprofit.
1. Decoding the Applications of Machine Learning: A Sector-Specific Perspective
To fully leverage ML, grasping its diverse models and applications is crucial. Below we classify ML models based on their respective real-world or potential applications in the nonprofit realm:
- Descriptive Models: These models illuminate past behaviors and trends. For example, the World Wildlife Fund (WWF) utilizes descriptive analytics to understand historical patterns in wildlife populations, deforestation, illegal trade, and many more.
- Diagnostic Models: These delve into data to unearth causes behind events or behaviors. In healthcare, organizations like Doctors Without Borders employ diagnostic analytics to identify factors driving health crises in remote regions.
- Predictive Models: By forecasting future events using historical data, these models aid in proactive planning. The Red Cross, for example, uses predictive analytics for disaster response, anticipating the impact of natural calamities on vulnerable communities.
- Prescriptive Models: Here, actionable suggestions are provided for optimal future outcomes. Educational nonprofits, like Khan Academy, leverage prescriptive analytics to tailor learning experiences, optimizing resource allocation.
- Generative Models: These models can create new synthetic data instances. Nonprofits harness them for innovative fundraising campaigns and structuring communication strategies, enhancing donor engagement, content generation, and more.
- Reinforcement Learning: Focusing on decision-making through trial and error, this approach can assist with optimizing resource distribution, much like how UNICEF allocates aid in emergency situations.
- Anomaly Detection: Identifying unusual patterns, crucial in fraud detection and maintaining transparency, is a key application in the financial management of nonprofits.
2. Navigating Development, Challenges, and Ethical Considerations
The nonprofit sector resolves the issues of the most vulnerable layers of society and, consequently, collects and analyzes their data. Thus, missteps in ML can profoundly affect these underserved communities. Therefore, ethical concerns and potential biases must be carefully addressed. The following lines summarize a few of these concerns:
- Data Privacy: Maintaining confidentiality, particularly in sensitive sectors like healthcare, is paramount. Thus, nonprofits must adhere to stringent data security standards.
- Bias and Fairness: ML models can inadvertently perpetuate bias and discrimination. Many strategies can be employed to mitigate biases and achieve fairness, including but not limited to, understanding the data's provenance and quality and the assumptions underpinning the models, engaging diverse stakeholders, equity assessments, and continuous monitoring.
- Continuous monitoring: Despite their reputation for adaptability, ML models require continuous monitoring in real-world applications to reflect the changes and trends in socio-economic factors, donor behaviors, and beneficiary needs, to mention a few. This can identify and mitigate biases that may not have been apparent or existent during the development phase.
- Resource Intensity: The development and maintenance of safe and effective ML models often demand significant computational resources and expertise, which is challenging for resource-strapped nonprofits.
Overall, nonprofits should strive for transparency by documenting the data sources, methodologies, and decision processes used in their ML systems. Additionally, nonprofits should consider establishing an ethics committee that includes not only data scientists but also representatives from the communities they serve. This diverse group can provide insights into the potential impacts of ML decisions and help maintain a focus on the organization's core values.
To become more familiar with these challenges, the "Blueprint for an AI Bill of Rights" published by the White House Office of Science and Technology Policy in October 2022 is a great starting point. This document stipulates five principles, elaborates on their importance, clarifies respective expectations from automated systems, and provides practical suggestions for implementation.
3. Conclusions
Machine learning is not just a technological tool; it's a pathway to enhanced efficacy and societal impact for nonprofits. Embracing ML responsibly can revolutionize operations and service delivery, marking a new era of data-driven philanthropy. To this end, nonprofits may consider the following suggestions:
- Integrating ML into organizational strategy and identifying relevant applications based on the organization’s mission and activities.
- Developing know-how’s for effective and safe collection and management of data and ensuring ethical use.
- Investing in organizational data literacy, implementing public datasets, and leveraging open-source tools and platforms through systematic training.
- Starting with pilot projects, adopting a culture of continuous performance evaluation, and using the obtained insights to iterate and improve future projects.
- And perhaps most importantly, building partnerships and collaborating with other nonprofits, academic institutions, or tech companies, as diversity in perspectives and experiences can be crucial to preventing failure.
For nonprofits, the journey into ML is not just a step forward – it's a leap towards a more effective, equitable, and impactful future.
4. Additional Resources
Please view this webinar recording during which we will provide you with the opportunity to learn more about the foundations of ML, explore its real-world applications, and grasp the basic guidelines for starting your first ML project while accounting for ethical consideration.